Overview #
Apache Paimon’s Architecture:
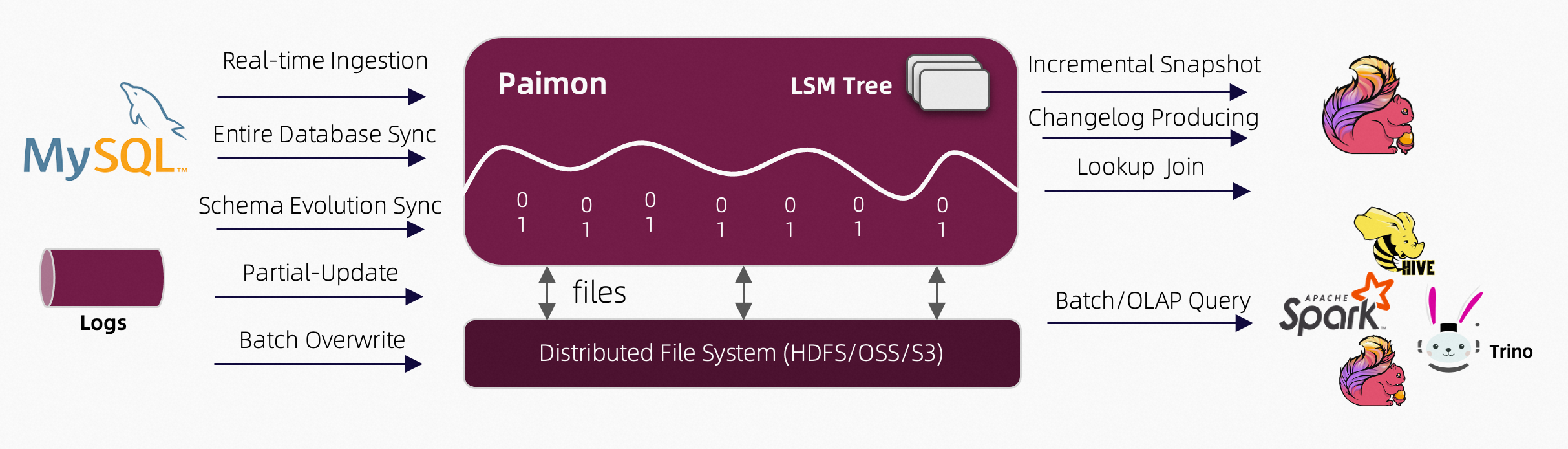
As shown in the architecture above:
Read/Write: Paimon supports a versatile way to read/write data and perform OLAP queries.
- For reads, it supports consuming data
- from historical snapshots (in batch mode),
- from the latest offset (in streaming mode), or
- reading incremental snapshots in a hybrid way.
- For writes, it supports
- streaming synchronization from the changelog of databases (CDC)
- batch insert/overwrite from offline data.
Ecosystem: In addition to Apache Flink, Paimon also supports read by other computation engines like Apache Hive, Apache Spark and Trino.
Internal:
- Under the hood, Paimon stores the columnar files on the filesystem/object-store
- The metadata of the file is saved in the manifest file, providing large-scale storage and data skipping.
- For primary key table, uses the LSM tree structure to support a large volume of data updates and high-performance queries.
Unified Storage #
For streaming engines like Apache Flink, there are typically three types of connectors:
- Message queue, such as Apache Kafka, it is used in both source and intermediate stages in this pipeline, to guarantee the latency stay within seconds.
- OLAP system, such as ClickHouse, it receives processed data in streaming fashion and serving user’s ad-hoc queries.
- Batch storage, such as Apache Hive, it supports various operations
of the traditional batch processing, including
INSERT OVERWRITE
.
Paimon provides table abstraction. It is used in a way that does not differ from the traditional database:
- In
batch
execution mode, it acts like a Hive table and supports various operations of Batch SQL. Query it to see the latest snapshot. - In
streaming
execution mode, it acts like a message queue. Query it acts like querying a stream changelog from a message queue where historical data never expires.